


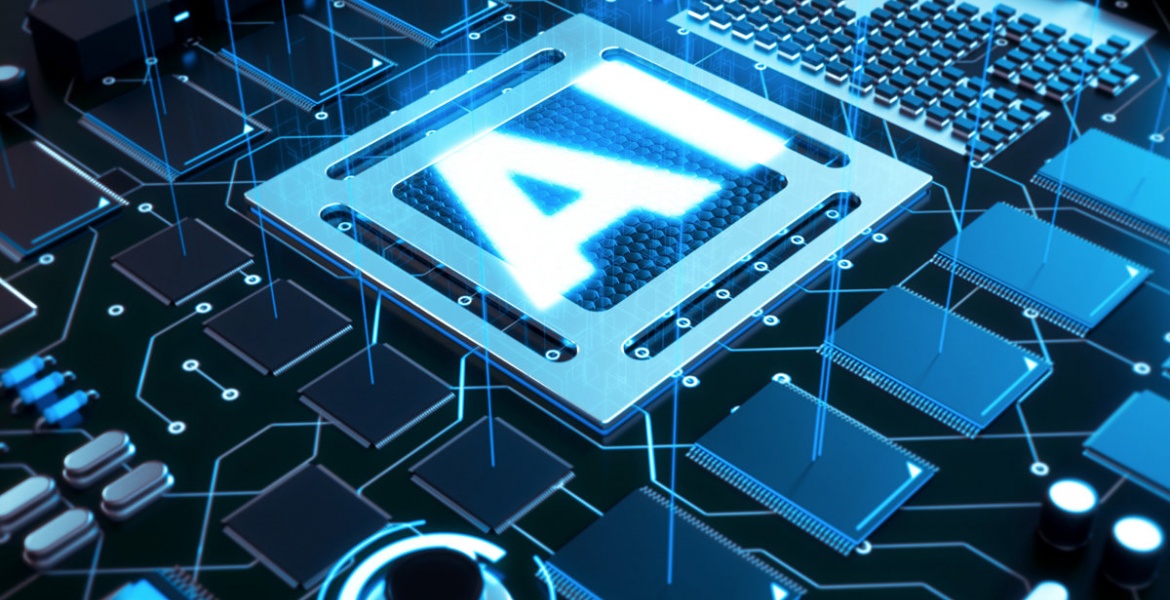
Attentional autoencoder for weighted implicit collaborative filtering
Content
Authors: Hoang-Vu Dang, Dung Ngo
Abstract: Many recommender systems operate on transactional data, of which the most widely available measure is the level of interaction between users and items. In real-world applications the recommendation process is often further divided into two stages: candidate generation, which selects a number of suitable items from the whole inventory, and ranking, which determines the order of suitability among the candidates. In this paper we define two separate tests to represent the candidate generation and ranking tasks, and evaluate a diverse set of old and new collaborative filtering methods on these tests. The results on three different datasets show that model accuracy on the two tasks do not correlate strongly with each other, thus in practical applications the model choice for each task should be considered independently. Furthermore we introduce an attentional autoencoder for implicit recommendation, based on the Autorec and Transformer architectures, trained with a variational objective. The proposed model yields consistently superior results to the other evaluated methods in both the candidate generation and ranking tasks.
Published in: CIIS 2019: Proceedings of the 2019 2nd International Conference on Computational Intelligence and Intelligent Systems
Date of Conference: November 2019
Conference Location: Bangkok Thailand

Do you need a workthrough of our platform? Let us know
Do you need a workthrough of our platform? Let us know
Cập nhật mọi tin tức mới nhất về công nghệ AI
Đăng ký nhận bản tin của FPT.AI để được phủ sóng mọi xu hướng công nghệ, câu chuyện thành công và phân tích của chuyên gia.