As an advanced product of Artificial Intelligence, Intelligent Agents (abbreviated as IA) were created to address the urgent need for automation and efficient decision-making in the era of exploding digital data. So, what exactly is IA, and why is it a core technology helping businesses stay ahead in the digital age? Let’s explore the role of Intelligent Agents and their differences from AI Agents in this article with FPT.AI.
What Is an Intelligent Agent?
An Intelligent Agent (IA), or bot (short for robot), is a computer program capable of making decisions or performing services based on its environment, user input, and its own experience. Below are the key characteristics of an Intelligent Agent:
- Autonomy: Intelligent Agents can operate independently without constant human intervention.
- Perception: Similar to humans using their senses, Intelligent Agents utilize sensors to gather information and process raw data from their environment, such as microphones and cameras in self-driving cars.
- Information Processing: Once an agent perceives its environment, it uses complex algorithms to analyze data, search for relevant information in databases, and determine the best course of action. This decision-making process is akin to a chess player quickly evaluating all possible moves.
- Action: Intelligent Agents interact with their environment through components like robotic fingers or wheels, while output devices like speakers and screens provide feedback to users.
- Learning and Adaptation: Intelligent Agents can learn from experience and adapt to changes in their environment rather than simply following a static set of rules. Each action provides valuable data to refine decision-making: positive outcomes encourage similar future actions, while poor results drive adjustments in the agent’s approach.
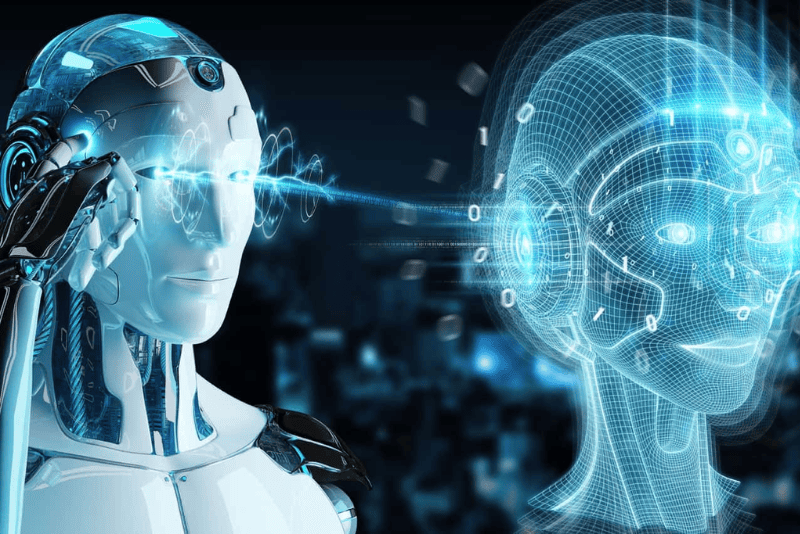
>>> Explore: What is Generative AI? Trends in Applying GenAI from 2024 to 2027
Types of Intelligent Agents
Intelligent Agents play a critical role in optimizing operations and enabling automated decision-making. Here are some types of Intelligent Agents categorized by their functionality and capabilities:
Simple Reflex Agents
Simple Reflex Agents operate based on the current state and disregard past events. Their responses rely on the Event-Condition-Action (ECA) rule, where a user initiates an event, and the agent references a list of pre-programmed rules and outcomes. For instance, An emergency shutdown system in a chemical plant. When detecting hazardous gas levels, the system immediately halts production without analyzing past events, ensuring damage is minimized instantly.
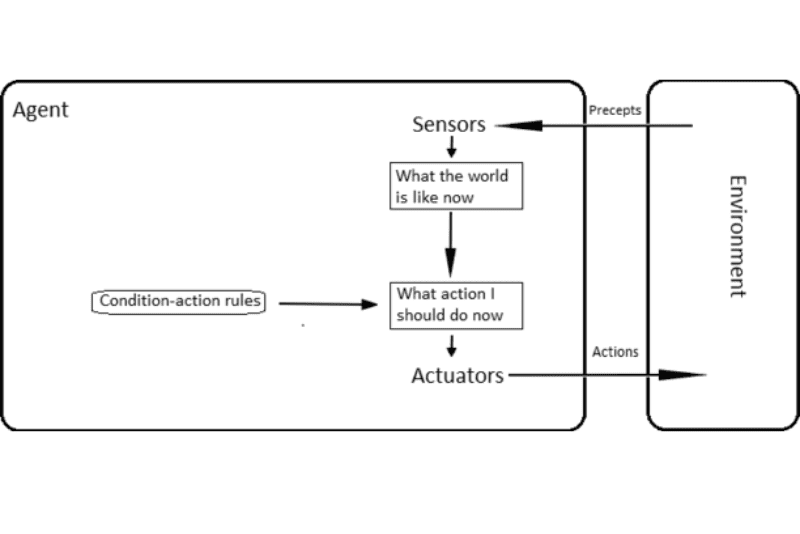
Model-Based Reflex Agents
These agents function similarly to Simple Reflex Agents but have a deeper understanding of their environment. Instead of reacting solely to the current situation, they rely on a pre-programmed world model, including the agent’s activity history. For example, a self-driving car approaching an intersection. It not only reacts to the current traffic signal but also considers the road layout, behaviors of other vehicles, and potential movements of pedestrians. This comprehensive view allows the car to navigate safely and efficiently.
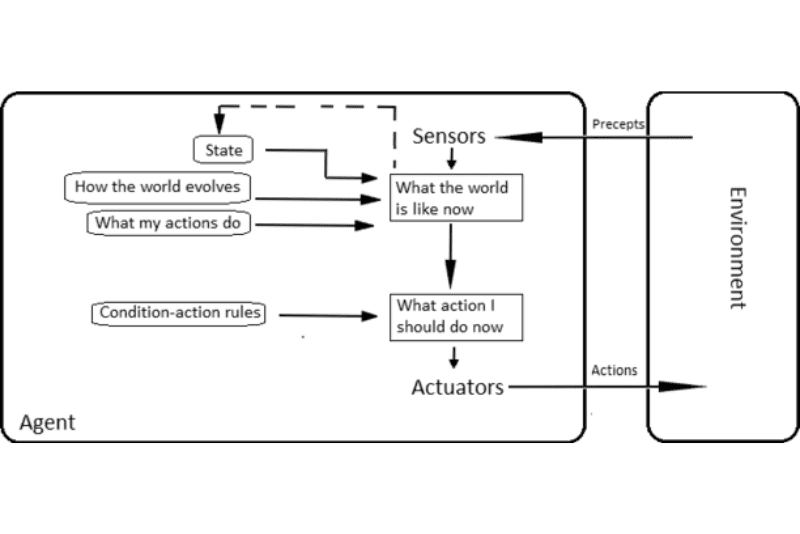
Goal-Based Agents
Goal-Based Agents expand on the information stored by including details about goals or desired outcomes. In healthcare, model-based agents can be applied to create personalized treatment plans. For instance, an AI system might analyze a patient’s medical records, genetic profile, and lifestyle factors to recommend a tailored treatment regimen, aiming for optimal health outcomes. This system considers long-term factors to ensure sustainable treatment effectiveness rather than just reacting to current symptoms.
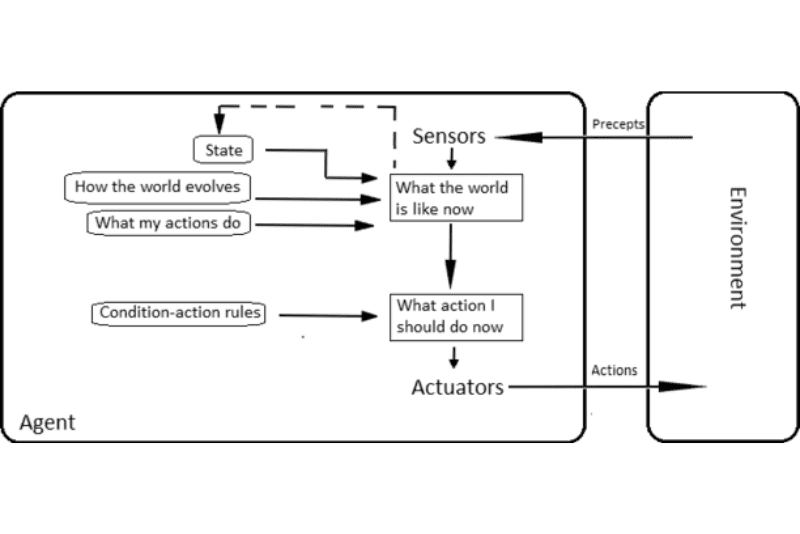
>>> Explore: What is a Multi Agent System (MAS)?
Utility-Based Agents
Utility-Based Agents operate similarly to goal-based agents but add a utility measurement to evaluate possible scenarios based on desired outcomes. These criteria can include success probabilities or required resources, allowing the agent to choose actions that maximize results.
In the financial industry, robo-advisors exemplify utility-based agents. These AI-powered investment tools aim not only for high returns but also consider factors like risk tolerance, liquidity needs, and long-term financial goals of clients. The agents craft personalized investment strategies to optimize each client’s financial portfolio, adapting to market changes while adhering to individual financial objectives.
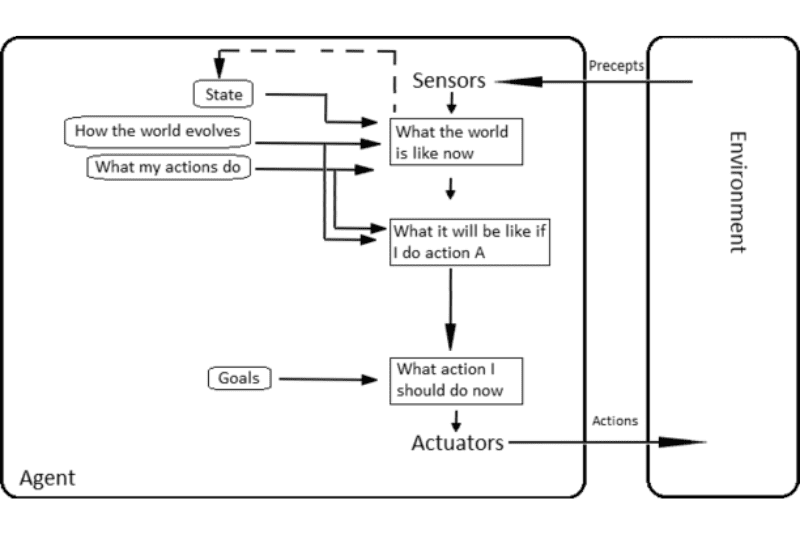
Learning Agents
Learning Agents have the capability to improve over time by using algorithms or additional learning mechanisms. These agents leverage feedback on performance metrics to refine processes and enhance their effectiveness gradually.
Recommendation systems on platforms like Netflix or Spotify are classic examples of Learning Agents. These systems analyze users’ viewing or listening habits, learn from their preferences, and adjust recommendations over time. As users continue to interact, the systems become smarter and deliver increasingly relevant and personalized content suggestion.
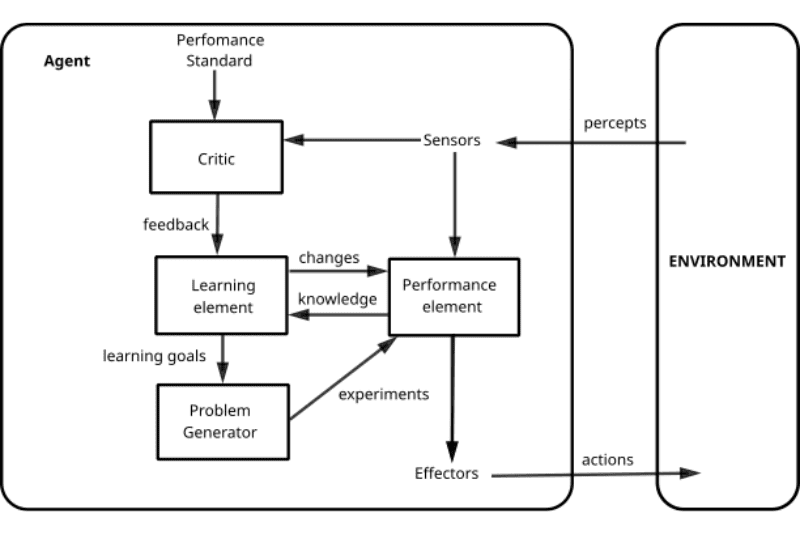
Differences Between Intelligent Agents and AI Agents
Intelligent Agents (IAs) are often confused with AI Agents due to their similar capabilities in gathering information, making decisions, and executing actions without direct human intervention. However, they differ significantly in autonomy, adaptability, and application scope.
Specifically, AI Agents focus on simulating human cognitive processes through predefined algorithms and rules. They primarily exist within computer systems and are widely used in customer service, gaming, and autonomous vehicles.
On the other hand, Intelligent Agents are a broader concept encompassing AI Agents. They can exist as physical or virtual entities and go beyond algorithms by interacting with environments, adapting to changes, and operating based on specific goals. IAs are commonly applied in autonomous robotics, smart home systems, industrial automation, and traffic management.
Challenges in Deploying Intelligent Agents
While Intelligent Agents have rapidly evolved, their implementation in real-world scenarios brings several challenges for businesses:
- Privacy and Data Security: Intelligent Agents process large amounts of data to function effectively. For instance, a customer support IA may need access to purchase histories and personal information to provide accurate recommendations. Companies must ensure that personal data is securely protected to avoid breaches or unauthorized use.
- Ethical Concerns: When Intelligent Agents take on critical decision-making roles, such as recruitment, they may inadvertently replicate biases embedded in historical data, favoring certain groups. Developers need to design and monitor these agents to ensure decisions are fair and aligned with societal ethical standards.
- Resource and Energy Requirements: As Intelligent Agents become more complex and powerful, they demand substantial hardware and energy resources. This raises environmental concerns and questions about AI accessibility. Advanced technologies may only be viable for resource-rich organizations, potentially widening the gap in AI adoption across industries and nations.
In conclusion, both AI Agents and Intelligent Agents play vital roles in enhancing automation and decision-making capabilities in modern technology systems. Their differences lie primarily in their level of perception and interaction with the environment. Understanding these agents helps apply them more effectively in fields such as industry, transportation, healthcare, and beyond.
FPT.AI hopes this article has provided you with a comprehensive overview of AI Agents and Intelligent Agents
>>> Read more about: What is Agentic AI? What make this technology surpass he Generative AI