AI Agents are shaping technology trends, with notable milestones such as the Google I/O 2023 event launching Astra or the emergence of GPT-4o. Large corporations are pouring billions of dollars into this technology to automate processes and optimize performance. In this article, FPT.AI will explore with you how AI Agents are helping businesses improve processes, enhance customer experience and optimize operations.
What are AI Agents? The Fundamental Components of an AI Agent
AI Agents are artificial intelligence systems that can interact with the environment and make decisions in the real world without any human guidance or intervention. They are personal assistants designed to simulate human intelligence, relying on AI technologies such as Machine Learning (ML) and Natural Language Processing (NLP) to process, analyze data and perform repetitive tasks based on user requests and desires.
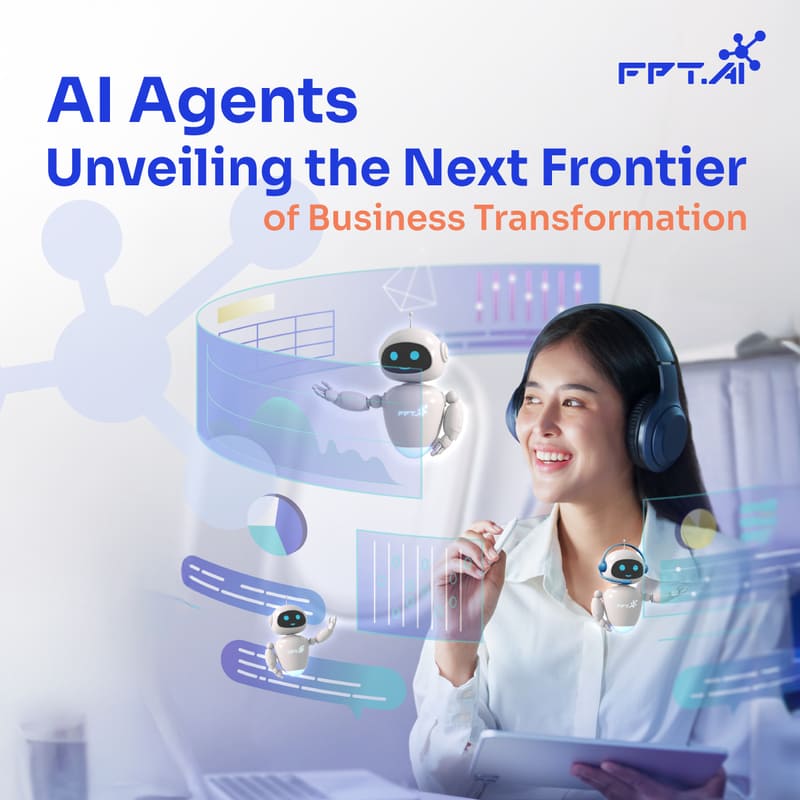
Key Features of AI Agents:
- Autonomy: AI Agents can work independently, make decisions, plan, and interact with external systems to achieve human-defined goals. For example, AI Agents in self-driving cars can automatically adjust speed, change lanes, stop, or adjust routes based on sensor data about road conditions and obstacles without human intervention.
- Continuous Learning: AI Agents can learn and adapt to changes in the environment to perform tasks better. For example, AI customer support assistants can improve the quality of responses by learning from millions of conversations to understand context and automatically provide more appropriate solutions to customers.
- Reactive and Proactive: AI Agents can predict and take actions before events occur. Nest Thermostat, a smart thermostat developed by Google, can learn from the user’s home temperature settings, reacting quickly when it detects sudden changes. If the temperature suddenly drops or rises too quickly, the system will adjust to maintain comfort levels and save energy.
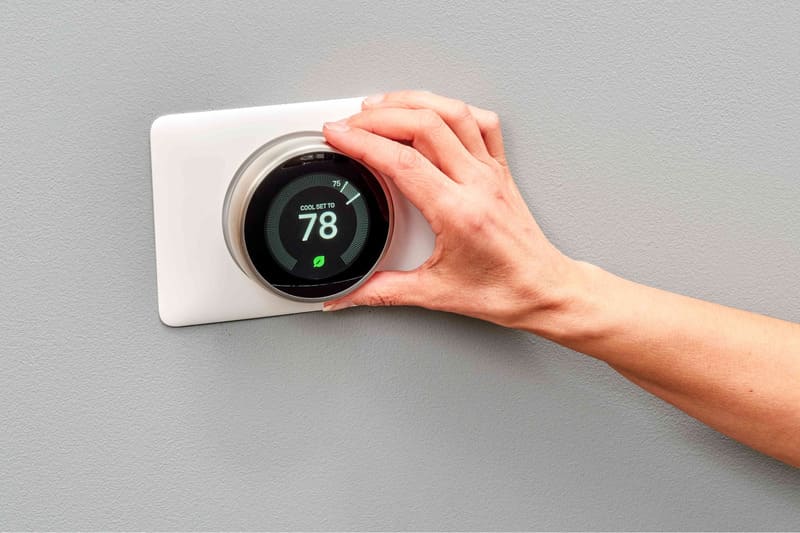
>>> EXPLORE: What Are Intelligent Agents? The Difference Between AI Agents and Intelligent Agents
How do AI agents work?
When receiving a command (goal) from the user (Prompt), AI Agents will plan and divide the goal into smaller, achievable tasks.
During the execution process, thanks to sensors, AI Agents will collect information (transaction data, customer interaction history) from many different sources (including external data sets, web searches, APIs and even other agents). During the collection process, AI Agents will continuously update their knowledge base, self-adjust and correct errors if necessary.
AI Agents’ processors will use algorithms, deep neural networks, machine learning models and artificial intelligence to analyze information and calculate the actions to take.
Throughout this process, the agents’ memory will continuously store information (such as a history of decisions made or rules learned) so that they can self-reflect, adjust their actions, and improve their performance over time. Finally, through the controllers (Actuators), AI Agents perform actions based on the decisions they have made. For robots, the controllers can be the parts that help it move or manipulate objects. For software agents, this can be sending information or executing commands on the system.
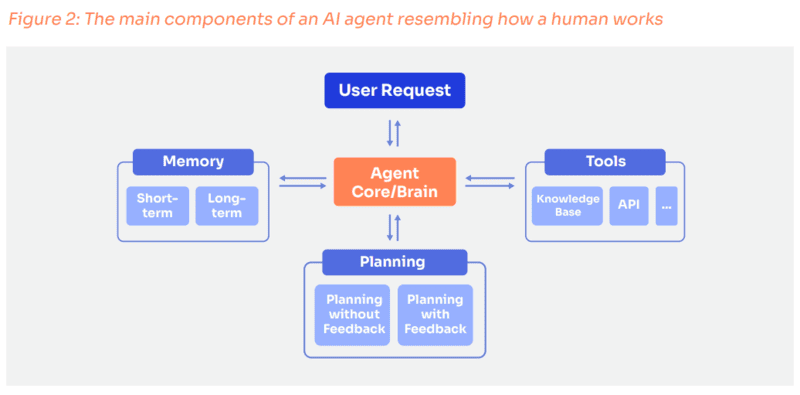
To illustrate this process, imagine a user planning their vacation. They ask an AI Agent to predict which week of the coming year will have the best weather for surfing in Greece. Since the large language model that underpins the agent is not specialized in weather forecasting, the agent must access an external database that contains daily weather reports in Greece over the past several years.
Even with historical data, the agent cannot yet determine the optimal weather conditions for surfing. Therefore, it must communicate with a surf agent to learn that ideal surfing conditions include high tides, sunny weather, and low or no rainfall.
With the newly gathered information, the agent combines and analyzes the data to identify relevant weather patterns. Based on this, it predicts which week of the coming year in Greece is most likely to have high tides, sunny weather, and low rainfall. The final result is then presented to the user.
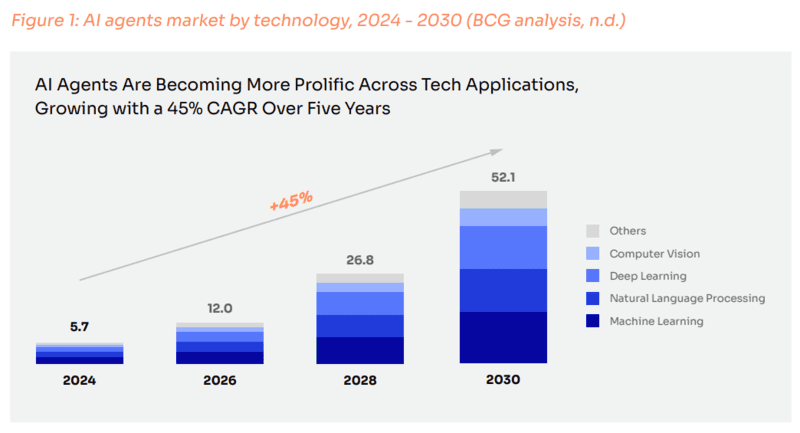
>>> READ NOW: What is a Multi Agent System (MAS)?
Differences between AI Agents and AI Chatbots
Below is a comparison table highlighting the distinctions between AI Agents and AI Chatbots:
Criteria | AI Agent | AI Chatbot |
Primary Purpose | Executes automated and independent tasks, often without human interaction | Interacts with humans, primarily for customer support or answering questions |
Automation Capability | Fully automates tasks without human intervention | Lacks full automation capability, relying on human interaction |
Human Interaction | May not require human interaction during operation | Mainly interacts with humans via text or voice |
Form | Can be software, physical robots, or smart home devices (e.g., vacuum robots, smart thermostats) | Primarily exists as text or voice-based interfaces (chat applications, chatbots, or virtual assistants) |
Task Processing Ability | Capable of handling complex tasks like automation and data-driven decision-making | Limited to basic questions and answers, with less capability for handling complex tasks |
Application Scope | Broad, applicable in various domains such as automation, medical diagnostics, and personal finance | Primarily in customer support, answering queries, and chat-based interactions |
Response Mechanism | Proactive and reactive to the environment, capable of planning and complex decision-making | Reacts based on predefined scripts and dialogue models, often limited in contextual understanding |
Learning Capability | Continuously learns and adapts based on feedback from the environment and other agents | Typically lacks continuous learning ability; updates are manual |
Context Processing | Deep understanding of context and emotions, capable of processing social signals and complex scenarios | Relies on fixed scripts, struggles with non-standard requests |
Example Applications | Robotic vacuum cleaners, business automation systems, smart thermostats, personal financial assistants | Customer support chatbots on websites, virtual assistants like Siri or Google Assistant |
>>> READ NOW: RPA vs AI Agents: Is RPA Still Relevant in the Age of AI?
Common Types of AI Agents
There are many different types of AI Agents, each suited to specific tasks and applications. Here are some common types of AI Agents:
- Simple Reflex Agents: Simple Reflex Agents operate on the “condition-action” principle and respond to their environment based on simple pre-programmed rules, such as a thermostat that turns on the heating system at exactly 8pm every night. The agent does not retain any memory, does not interact with other agents without information, and cannot react appropriately if faced with unexpected situations.
- Model-Based Reflex Agents: Model-Based Reflex Agents use their cognitive abilities and memory to create an internal model of the world around them. By storing information in memory, these agents can operate effectively in changing environments but are still constrained by pre-programmed rules. For example, a robot vacuum cleaner can sense obstacles when cleaning a room and adjust its path to avoid collisions. It also remembers areas it has cleaned to avoid unnecessary repetition.
- Goal-Based AI Agents: Goal-Based Agents are driven by one or more specific goals. They look for appropriate courses of action to achieve the goal and plan ahead before executing them. For example, when a navigation system suggests the fastest route to your destination, it analyzes different paths to find the most optimal one. If the system detects a faster route, it updates and suggests an alternative route.
- Utility-Based Agents: Utility-Based Agents can optimize outcomes by using a utility function to measure how useful each action is based on criteria such as progress toward a goal, time, or complexity of the process. For example, a navigation system considers factors such as fuel efficiency, reduced travel time, and toll costs to select and recommend the most convenient route for the user.
- Learning Agents: Learning Agents learn from interactions and feedback from the environment or the user to improve performance over time. Learning occurs automatically, allowing the agent to operate effectively in unfamiliar environments. For example, e-commerce websites using Learning Agents are able to understand user needs and preferences to provide personalized product recommendations.
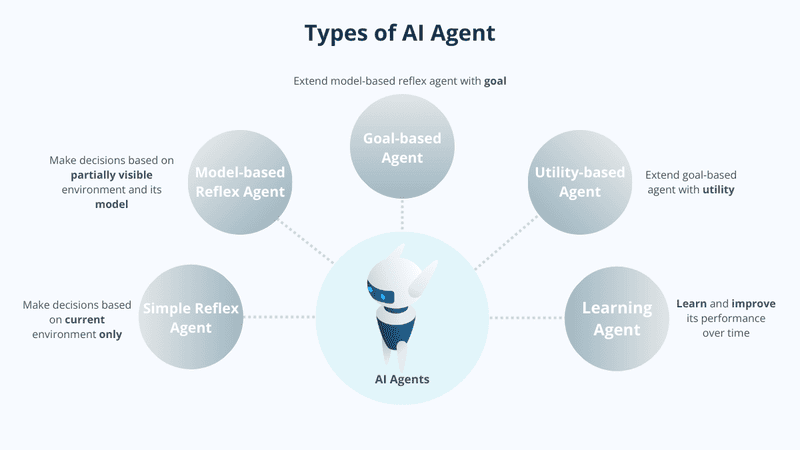
>>> EXPLORE: What is an LLM Agent? How it works, advantages, and disadvantages
Is ChatGPT an AI Agent?
ChatGPT is not an AI Agent, but rather a large language model designed to perform tasks by generating human-like responses based on input received. This AI assistant cannot perform actions or set goals on its own, but must rely on user instructions. For example, if you ask ChatGPT to write an email, it can generate content but cannot send the email itself or determine whether sending the email is the best course of action.
ChatGPT also does not have the ability to directly interact with systems or adjust its behavior based on real-time feedback. While plugins or frameworks can improve ChatGPT’s functionality, these additions do not make it a true Agent.
Additionally, while advanced AI Agents can learn from interactions, improve performance over time, and use memory to inform future actions. ChatGPT does not retain memory between sessions, unless specifically programmed into certain applications.
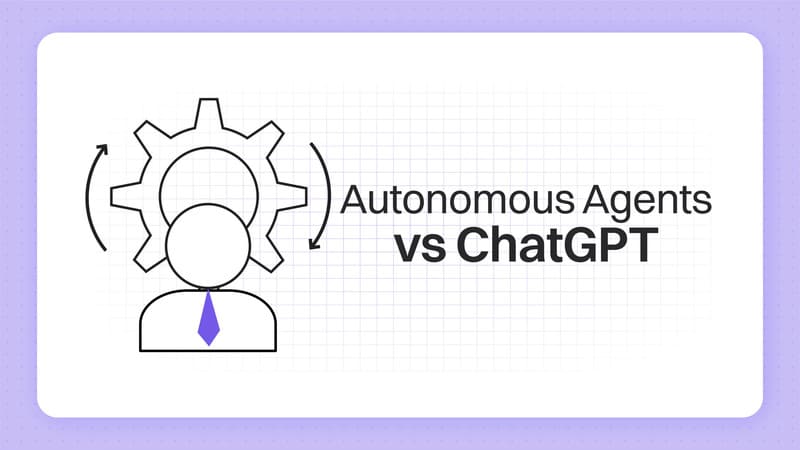
What are the outstanding benefits of using AI Agents?
AI Agents help businesses deliver a consistent experience to customers across multiple channels, with the following 4 outstanding benefits:
- Improve productivity: AI Agents help automate repetitive and time-intensive tasks, freeing up human resources from manual work so that businesses can focus on more strategic, creative and high-value initiatives, fostering innovation. For more complex issues, AI Agents can intelligently escalate cases to human agents. This seamless collaboration ensures smooth operations, even during periods of high demand.
- Reduce costs: By optimizing processes and minimizing human errors, AI personnel help businesses cut operating costs. Complex tasks are handled efficiently by AI Agents without the need for constant human intervention.
- Make informed decisions: AI Agents use machine learning (ML) technologies to help managers collect and analyze data (product demand or market trends) in real time, making faster and more accurate decisions.
- Improve customer experience: AI agents significantly enhance customer satisfaction and loyalty by offering round-the-clock support and personalized interactions. Their prompt and precise responses effectively address customer needs, ensuring a smooth and engaging service experience. Lenovo leveraged AI agents to streamline product configuration and customer service, integrating them into key systems like inventory tracking. By building a knowledge database from purchase data, product details, and customer profiles, AI agents help Lenovo cut setup time from 12 minutes to 2 minutes, boosting sales productivity and customer experience. This led to a 12% improvement in order delivery KPIs (within 17 days) and generated $5.88 million in one year, according to Gartner.
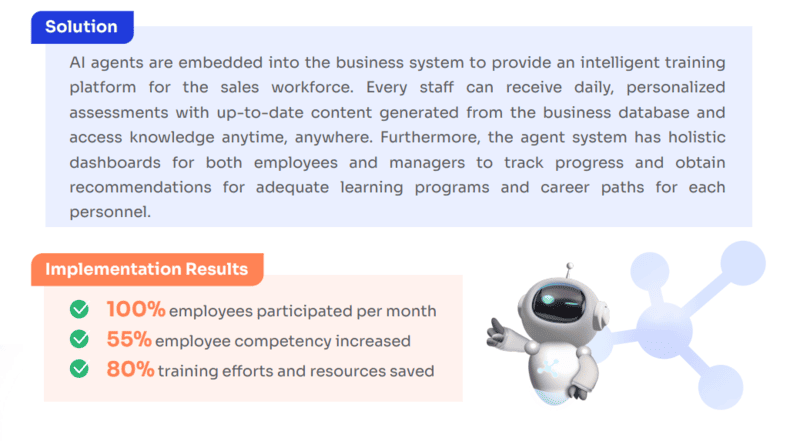
>>> Read more about: What is Generative AI? Trends in Applying GenAI from 2024 to 2027
Practical Applications of AI Agents
Imagine a future workplace where every employee, manager, and leader not only works together, but is also equipped with a team of AI teammates to support them in every task and at every moment of the workday. With these AI teammates, we will become 10x more productive, achieve better results, create higher quality products, and of course, become 10x more creative.
You may be wondering, “When will this future come?” The answer from FPT is: The future is now. Here are four stories that demonstrate how AI is already impacting businesses.
Revolutionizing Insurance Claims Processing
Imagine you go to the hospital for a health check-up, buy medicine, and file an insurance claim. Typically, the insurance company’s document processing will take at least 20 minutes. With integrated AI Agents, insurers can process all documents through rapid assessment tools, risk assessment tools, and fraud detection tools, returning results in just 2 minutes.
This represents an incredible leap in productivity, improving the customer experience and creating new competitive value for the business.
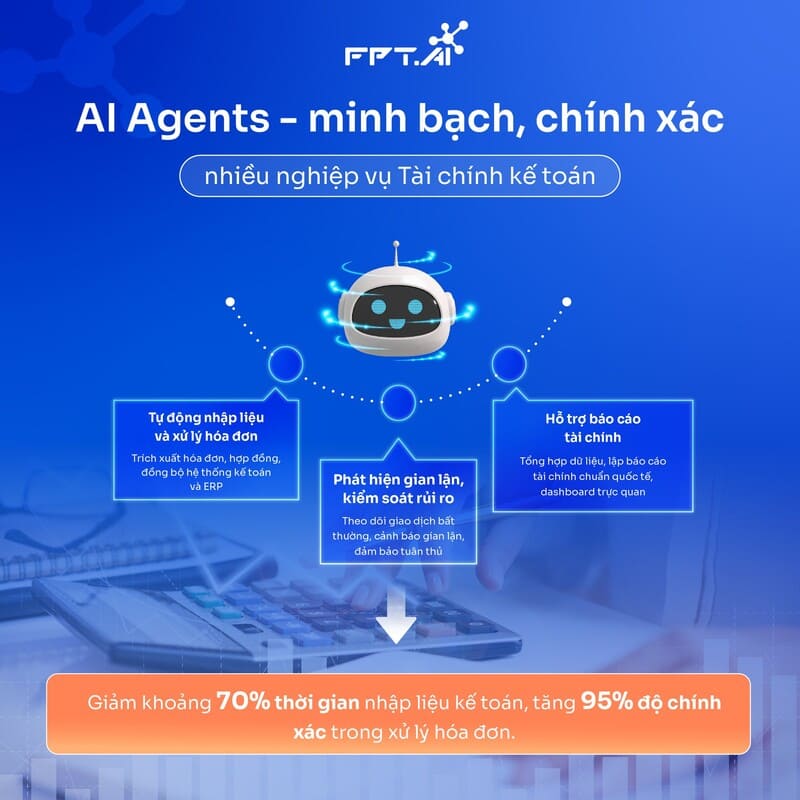
Transforming the Customer Contact Center
The second story focuses on customer service. Several FPT.AI customers have deployed AI systems for inbound and outbound communications. These systems provide human-like customer support, handling requests, resolving issues, and providing excellent service.
For some customers, AI Agents are now handling 70% of customer requests, completing 95% of received tasks, and achieving a customer satisfaction rating of 4.5/5. Currently, FPT’s customer service AI Agents manage 200 million user interactions per month.
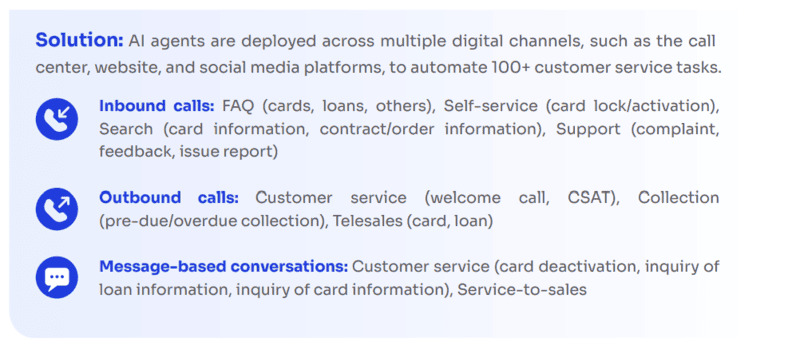
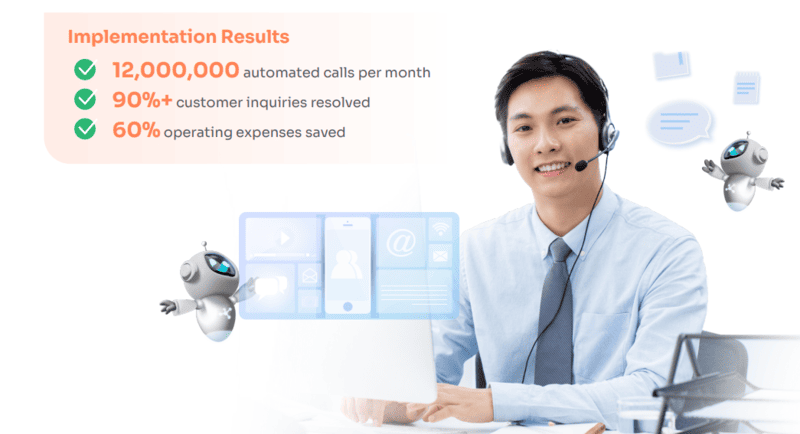
Empowering pharmacists with AI Mentor
At Long Chau, the largest pharmacy chain in Vietnam, more than 14,000 pharmacists work every day to advise customers. To ensure they stay updated with knowledge and work effectively, FPT.AI has developed an AI Mentor that interacts with more than 16,000 pharmacists across 2,000 pharmacies every day.
This AI Mentor identifies strengths and weaknesses, provides insights, and personalizes conversations to help them improve. The results are:
- Pharmacists’ competencies improved by 15%.
- Productivity increased by 30%.
Within the first nine months of the year, the pharmacy chain recorded a revenue growth of 62%, reaching VND 18.006 trillion, accounting for 62% of FRT’s total revenue and completing 85% of its 2024 plan. More importantly, we pride ourselves on helping pharmacists become the best versions of themselves while continuously improving.
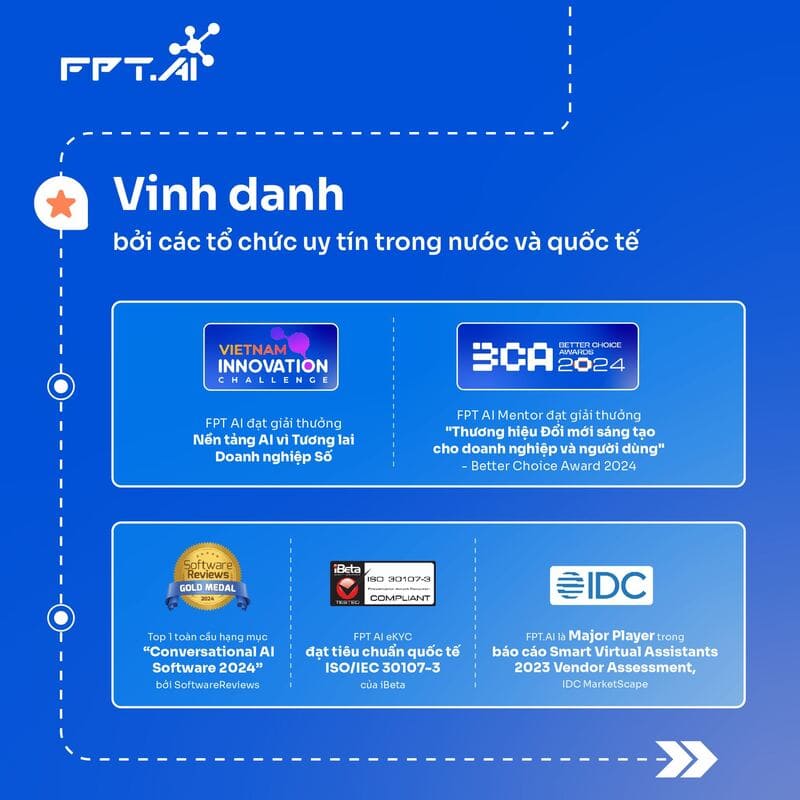
From a cost center to a profit center
FPT.AI’s AI Innovation Lab works with customers to identify opportunities, deploy pilots, and scale solutions. For example, one of our clients transformed their customer service center from a cost center to a profit center.
Using AI, they detected when customers were happy and immediately suggested appropriate products or services to upsell credit cards, cross-sell overdrafts, activate new customers to sign up, and reactivate existing customers. This approach helped the customer service center contribute about 6% of total revenue.
The four stories above are just a small part of the countless ways AI can transform businesses. AI, as a new competitive factor, is opening up a blue ocean of innovation. Every company and organization will need to reinvent their operations and build a strong foundation to compete in the future, leveraging the advances of AI.
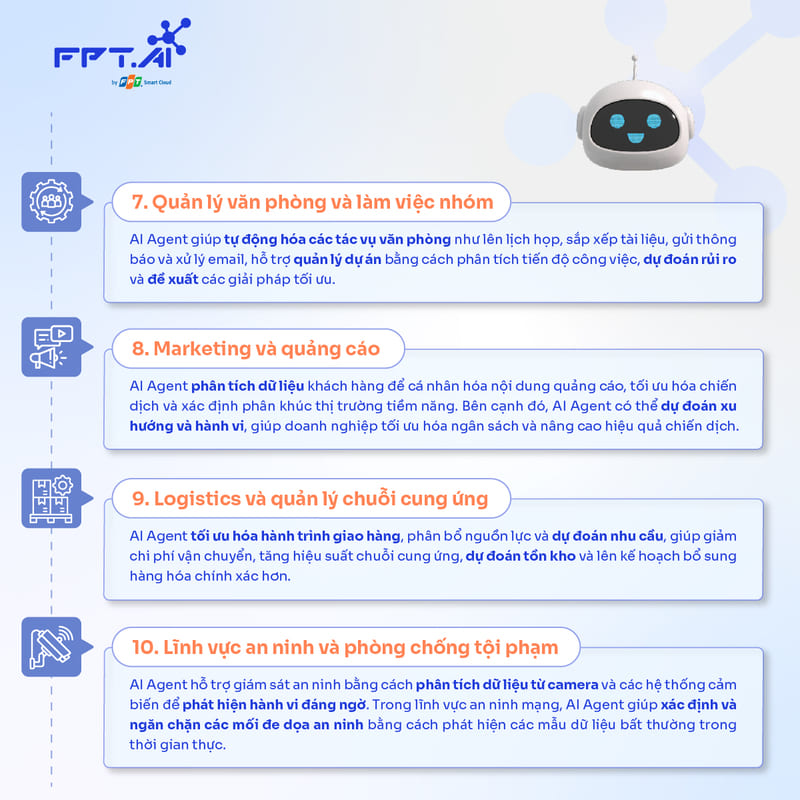
>>> EXPLORE: What is Agentic AI? The differences between Generative AI and Agentic AI
Challenges in Deploying AI Agents
AI Agents are still in their early stages of development and face many major challenges. According to Kanjun Qiu, CEO and founder of AI research startup Imbue, the development of AI Agents today can be compared to the race to develop self-driving cars 10 years ago. Although AI Agents can perform many tasks, they are still not reliable enough and cannot operate completely autonomously.
One of the biggest problems that AI Agents face is the limitation of logical thinking. According to Qiu, although AI programming tools can generate code, they often write wrong or cannot test their own code. This requires constant human intervention to perfect the process.
Dr. Fan also commented that at present, we have not achieved an AI Agent that can fully automate daily repetitive tasks. The system still has the ability to “go crazy” and not always follow the exact user request.
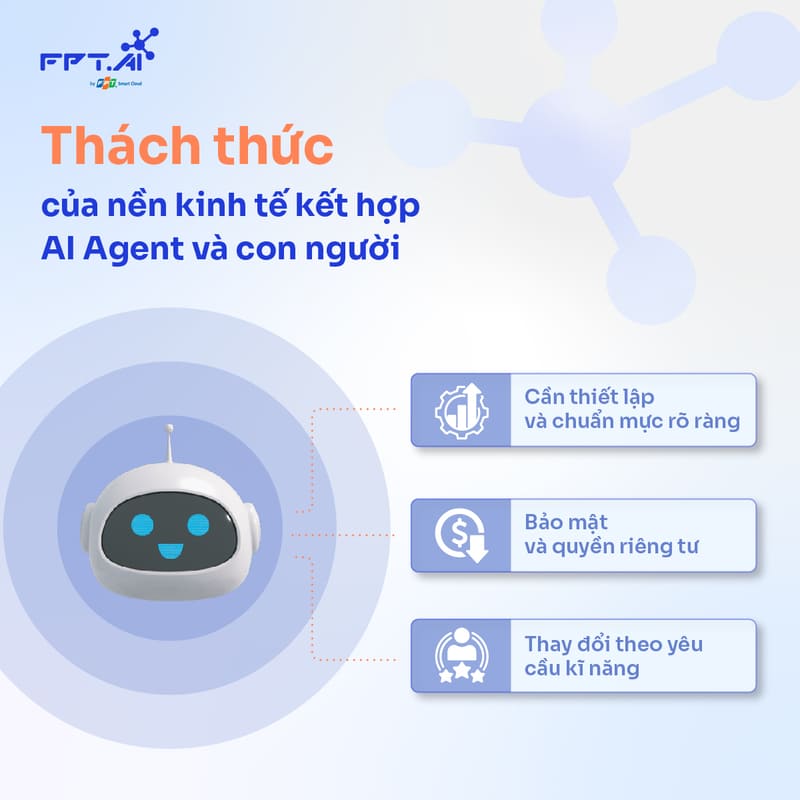
Another major limitation is the context window – the ability of AI models to read, understand, and process large amounts of data. Dr. Fan explains that models like ChatGPT can be programmed, but have difficulty processing long and complex code, while humans can easily follow hundreds of lines of code without difficulty.
Companies like Google have had to improve the ability to handle context in their AI models, such as with the Gemini model, to improve performance and accuracy.
For “physical” AI Agents such as robots or virtual characters in games, training them to perform human-like tasks is also a challenge. Currently, training data for these systems is very limited and research is just beginning to explore how to apply generative AI to automation.
>>>> EXPLORE: What is Data Leakage? How to Prevent Data Leakage when implementing Generative AI?
Continue writing the future with AI Agents with FPT.AI
In the digital economy, competition between companies and countries is no longer based solely on core resources, technology and expertise. Organizations, from now on, will need to compete with a new important factor: AI Companions or AI Agents.
It is expected that by the end of 2025, there will be about 100,000 AI Agents accompanying businesses in customer care, operations and production. Each AI Agent will undertake a number of tasks such as programming, training, customer care… Thanks to that, employees are more empowered, businesses increase operational productivity, improve customer experience, and make more accurate decisions based on data analysis.
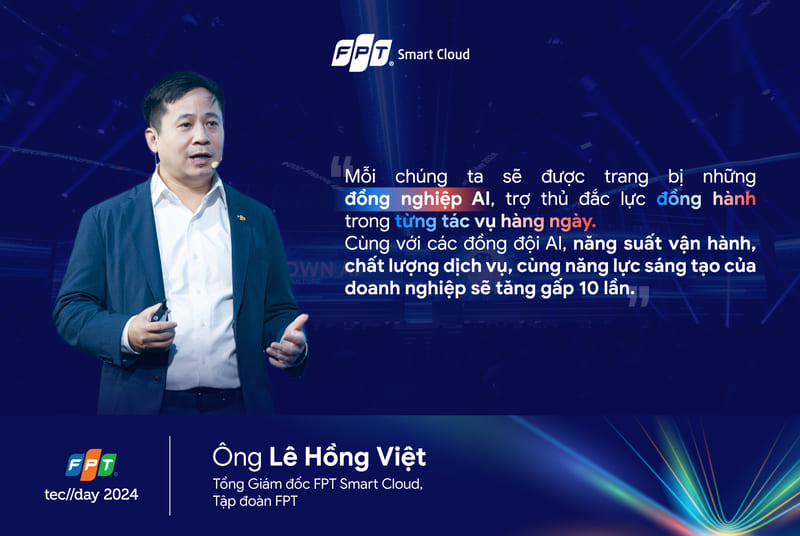
FPT AI Agents – a platform that allows businesses to develop, build and operate AI Agents in the simplest, most convenient and fastest way. The main advantages of FPT AI Agents include:
- Easy to operate and use natural language.
- Flexible integration with enterprise knowledge sources.
- AI models are optimized for each task and language.
Currently, FPT AI Agents supports 4 languages: English, Vietnamese, Japanese and Indonesian. In particular, AI Agents have the ability to self-learn and improve over time.
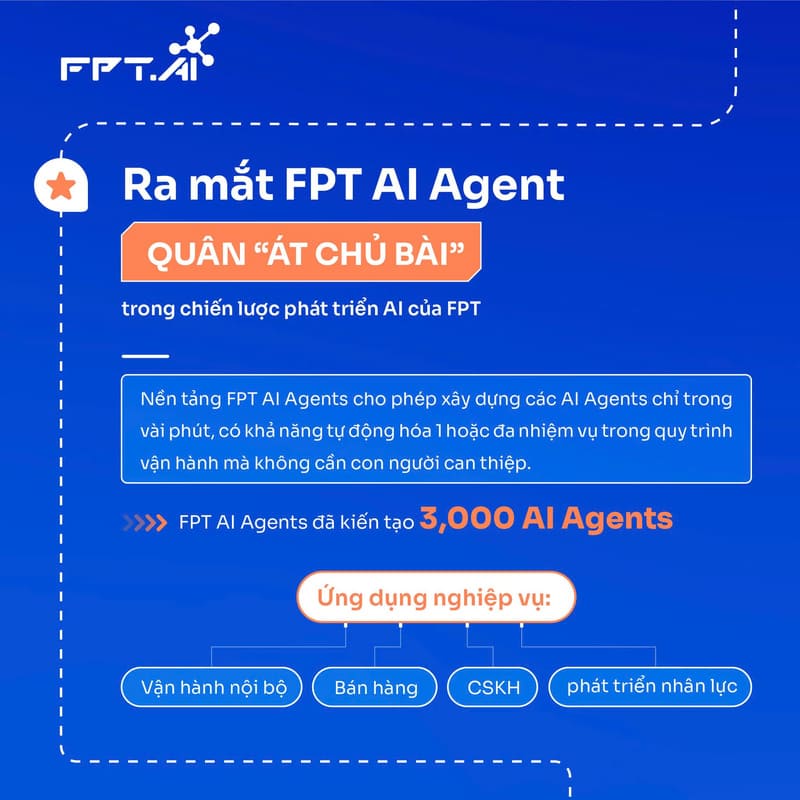
AI Agents are all operated on FPT AI Factory – an ecosystem established with the mission of empowering every organization and individual to build their own AI solutions, using their data, supplementing their knowledge and adapting to their culture. This differentiation fosters a completely new competitive edge among enterprises and extends to building AI sovereignty among nations.
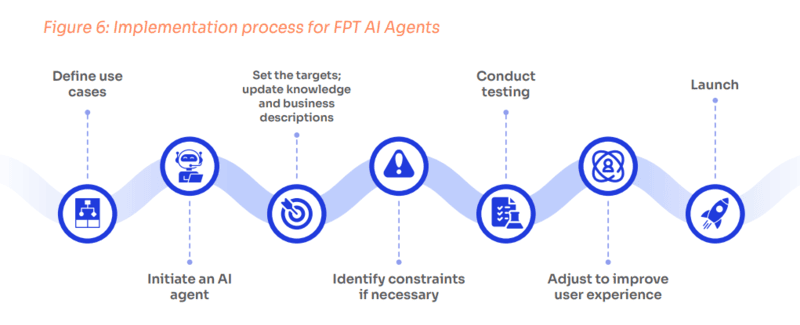
With more than 80 cloud services and 20 AI products, FPT AI Factory helps accelerate AI applications by 9 times thanks to the use of the latest generation GPUs, such as H100 and H200, while saving up to 45% in costs. These factories are fully compatible with the NVIDIA AI Enterprise platform and architectural blueprints, ensuring seamless integration and operation.
You can watch a demo of an AI Agent developed on the FPT AI Agents platform in the following video: